Top stories






More news


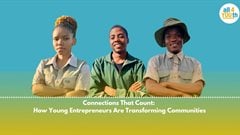




Marketing & Media
#YouthMonth: BET Software champions young minds with Robotics Clubs



















Calls for action with regard to combating the spread of fake news abound and social media sites and search engines including Facebook and Google have already taken measures to that effect. The latest technology is now being used to verify content and spot fakes. Of course, this is not always easy as fake news can actually mean several different types of information, noted Pierre-Habté Nouvellon, the CTO at Snipfeed, a California-based startup that has created an eponymous AI-based news and information recommendation engine.
While news asserting facts that are easily proved wrong is relatively easy to fight, another type of fakes – information that is presented as a fact but which is rather an opinion or an oversimplified answer to an intricate problem – tends to be much more vicious, he pointed out. Fake news can be spread through articles and videos with the latter being used to convey so-called deep fakes – videos (often depicting public figures) altered in a way that they look realistic but, in fact, show those people saying things they never said.
Deep fakes are still difficult to detect, but the issue is now getting a lot of experts’ attention. Meanwhile, machine learning is already very useful in spotting anomalies in articles and identifying content that looks suspicious, said Nouvellon who hopes that Snipfeed will become the main news source for young people and a platform for critical thinking. He added that fake news articles are often defined by a weird distribution of words and the use of a vocabulary that is slightly different from that used by journalists in high-quality journalistic news pieces.
For example, they usually feature many superlatives. “It is possible to train a machine learning model to classify articles based on the word distribution of those articles and get good results,” Nouvellon maintained. “However, this is not enough as very well written fake news pieces have a normal distribution of words,” he added. In his opinion, this is where checking the facts becomes crucially important – machine learning can help here by extracting the main fact or idea from a given article and comparing it with other sources.
A subset of artificial intelligence, machine learning consists in the study of algorithms and statistical models to perform certain tasks without explicit instructions. A machine learning model learns from the past to predict the future by relying on patterns and inference. It needs data that were generated in the past to be trained and the data used for training need to be similar to the data that the model will use for making predictions. “Trying to use a model trained on fake news from the Middle Ages to detect modern fake news would be a bad idea,” Nouvellon joked.
As a CTO, Mr Nouvellon’s role in building and deploying the mechanism used by Snipfeed was critical. Snipfeed, which he co-founded and provides daily and highly personalised snippets for its users, has developed an internal tool called Fakebuster to detect fake news articles and avoid recommending them to its users.
Snipfeed has been recognised by world-renowned accelerators such as Berkeley SkyDeck, the UC Berkeley startup accelerator, or The Refiners. As the CTO, Mr Nouvellon’s role in building and deploying the mechanism used by Snipfeed was critical. Nouvellon said that while the quality of a particular article is a very subjective feature, Fakebuster still tries to assess it by applying a number of underlying assumptions such as these: A serious article gives facts that can be verified true with other “serious” sources, and a serious article’s headline should be related to the text of the articles (which is usually not the case with clickbait).
Fakebuster uses three sub-scores – a fact-checking score, a writing style score and a headline score – to verify the reliability of facts, evaluate if an article meets the linguistic standards of a well-written piece and assess how well the headline reflects a given article’s content. A final score is then made by linearly combining the three above-mentioned sub-scores. “We use the final score to filter out articles,” Nouvellon revealed. The Snipfeed engine’s future performance as far as attracting new users is concerned will show whether its fake news busting tool works.