A Rutgers study has found that gender bias and stereotypes corresponding to certain occupations are prevalent on digital and social media platforms.
The study, published in the Journal of the Association for Information Science and Technology, finds that online images of men and women in four professions – librarian, nurse, computer programmer and civil engineer – tend to represent and reinforce existing gender stereotypes.
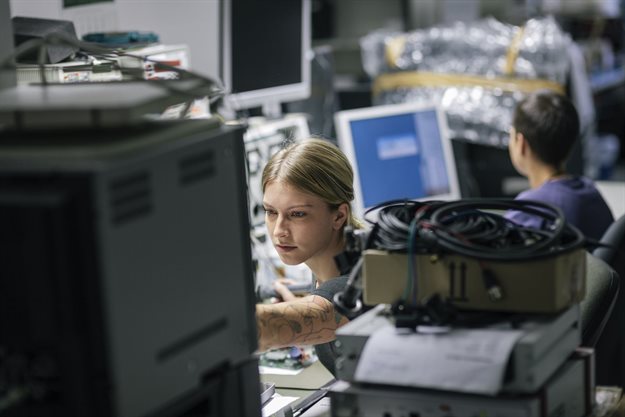
Image source: Gallo/Getty Images.
Rutgers researchers analysed search results for images of people in each of the four occupations on four digital media platforms: Twitter, NYTimes.com, Wikipedia and Shutterstock. They also compared the search results to the gender representation of each occupation as per the US Bureau of Labor Statistics.
The results showed gender stereotypes and biases to be prevalent. Women were over-represented as librarians and nurses and under-represented as computer programmers and civil engineers, especially when the collection and curation of content is largely automated by an algorithm, such as on Twitter.
Direct content curation
However, on platforms where individuals can generate and curate content more directly, such as the NYTimes.com and Shutterstock, stereotypes were more likely to be challenged. Search results of NYTimes.com, for example, produced images of civil engineers who are women, and nurses who are men, more often than would be expected given their representation in the Labor Statistics.
“More direct content curation will help counter gender stereotypes,” said Vivek Singh, an assistant professor of library and information science at Rutgers’ School of Communication and Information.
While women generally tend to be under-represented in male-dominated professions on digital media platforms, Singh noted some progress toward equity in the gendered presentation of images from 2018 to 2019. For instance, more women were shown in images for male-dominated professions on Twitter in 2019 than in 2018.
“Gender bias limits the ability of people to select careers that may suit them and impedes fair practices, pay equity and equality,” said co-author Mary Chayko, a sociologist and interdisciplinary teaching professor at the School of Communication and Information. “Understanding the prevalence and patterns of bias and stereotypes in online images is essential and can help us challenge and hopefully someday break these stereotypes.”
The researchers said that the study could help prevent biases from being designed into digital media platforms, algorithms and artificial intelligence software, and while human beings indeed construct algorithms, the study’s results may help content creators and platform designers identify whether algorithm‐heavy or human‐heavy curation may be better suited to a task.
The study was co-authored by Raj Inamdar, a research associate at Rutgers’ Behavioral Informatics Lab and Diana Floegel, a doctoral student at Rutgers’ School of Communication and Information.