Opinion mining the next level in social analytics...
A wealth of unstructured opinion data exists online – data that was, until now, unusable. Such data, moreover, is growing all the time. We add to it daily when we talk about our likes and dislikes on social media and elsewhere on the web. We do so in an honest and unsolicited manner, expressing our true feelings towards a brand, a politician, or a global event. The challenge of analysing these data points on a massive scale has created a new science – that of opinion mining.
Opinion mining: The basics
In the broadest terms, opinion mining is the science of using text analysis to understand the drivers behind public sentiment.
All text is inherently minable. As such, while social media may be an obvious source of current opinion, reviews, call centre transcripts, web pages, online forums and survey responses can all prove equally useful.
Sentiment analysis versus opinion mining
Whereas sentiment analysis – a predecessor to the field of opinion mining – examines how people feel about a given topic (be it positive or negative), opinion mining goes a level deeper, to understand the drivers behind why people feel the way they do.
Why mine?
Individual opinions are often reflective of a broader reality. A single customer who takes issue with a new product’s design on social media likely speaks for many others. The same goes for a member of the public who takes to a political campaigner’s web page to praise or criticise the policies proposed.
Gather enough opinions – and analyse them correctly – and you’ve got an accurate gauge of the feelings of the silent majority. This relates not only to how people feel, but the drivers underlying why they feel the way they do.
What is driving the sentiment?
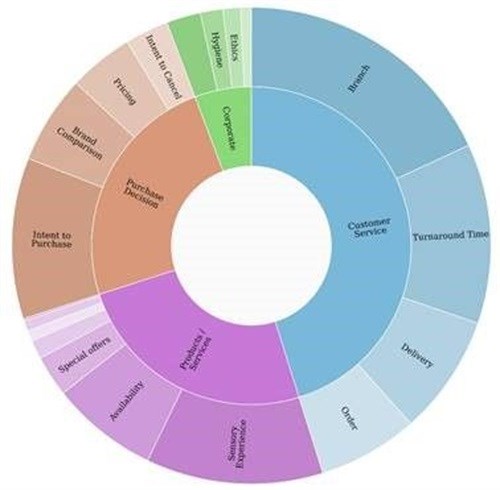
BrandsEye topic wheel of a global fast food chain
By understanding what is driving the sentiment, opinion data can be used to expose critical areas of strength and weakness. This data allows executives to make the targeted, strategic overhauls needed to reinvigorate profitability or reclaim slipping market share.
Within the public sector this same data can be used to build strategies and campaigns that resonate with the electorate and react to voters’ changing needs. By isolating the specific, topic-level drivers of positive and negative sentiment, opinion mining allows for the development of an incredibly deep level of social insight – a window into how people really think and feel.
By analysing conversations for both sentiment and the topics driving that sentiment, a retail bank might discover, that of customers’ criticisms, queue length and waiting times feature uppermost.
A fast food chain might be interested to know that relative to their closest competitor, many consider their portion size too small, though their friendly customer service is a plus.
Yet it’s possible to delve deeper still. The topic of customer service could be further broken down into the sub-categories of turnaround time, order correctness and delivery time. The business may have great in-store turnaround, but fail when it comes to deliveries. Knowing which issue to target – and why – is key.
Computing versus the crowd: The science of sentiment
The quantity of minable text available is absolutely massive. Given the volume, a degree of computational brute force is necessary. With this in mind, artificial intelligence can be used to good effect to carry out a large part of the deciphering grunt work.
In a nutshell, artificial intelligence is used to break down content into component parts (nouns, verbs, emotive words, etc.) to develop an understanding of its author’s feelings. For many text analysis tasks – but particularly those which are large-scale and the text requiring analysis is relatively standardised – AI can prove an excellent approach.
Machine learning approaches
The area of machine learning dedicated to making sense of the written word is known as natural language processing.
There are various approaches, including:
- Supervised machine learning, which make use of structured data and/or human annotation (i.e. a set of ‘answers’) from which machines learn in order to make future inferences;
- Semi and unsupervised machine learning, under which no guidance is supplied, and machines must learn to interpret unstructured data with minimal or no guidance;
- Deep learning. These are complex, multi-level systems. Recent advances in the sphere have shown promising results.
Buckets and basics
Let’s start with a simple example: an online author tweets about a car brand, “Love the new model – great ride!”. The first step in making sense of this text is to establish the comment’s overall polarity. In other words, how the author feels about the topic at the broadest level. One of the simplest approaches to determining polarity is by using the presence of certain keywords to assign a comment to one of three buckets: positive, negative or neutral.
On detecting a word such as love – or equally, like, hate, dislike, enjoy, etc. – a textual analysis algorithm would classify the comment that possesses it accordingly. Unfortunately, understanding language is not always this simple. This approach also offers little nuance or indication of why the author felt that way.
Sophistication and style
“Likes” differs from “adores” as much as dislikes “differs” from “abhors”. The issue is one of emotional intensity. More sophisticated AI text analysis approaches can account for such differences. To capture variations in sentiment strength, rating scales are commonly used. A scathing comment, “Absolutely hated the new flavour! Would not recommend!”, might be assigned the maximum negative value of -5. Its praise-filled opposite, “Adore it! Delicious!”, might score +5. Neutrality, “Okay flavour. Ho-hum”, scores a zero.
Improving accuracy with humans
AI-driven approaches, though increasingly sophisticated, remain imperfect. People communicate in complex ways: slang, vernacular, misspellings, figurative language and long, meandering sentences can all limit machine interpretation. The offhand, unstructured dialogue that fills the web, in particular, is culturally and socially complex.
The same things that make language lively and human – humour, slang, innuendo, sarcasm, colloquialisms, figures of speech – are exactly the same things that confound machines. Adding a layer of human insight can significantly improve the accuracy with which these nuances can be interpreted. An emerging approach is to use crowd-sourcing technology to add human insight to data at scale. This involves sending text to real people – the ‘crowd’ – and asking them to label it, then checking results against other crowd members to achieve maximum accuracy. If this data is fed back to an algorithm, it can also be used to teach machines to better interpret data based on human understanding.
There are three main approaches to crowd analysis:
- Manual coding – Using internal resources to look at each data point and manually label it for sentiment and topics. This method can be very accurate but is time consuming and lacks scalability.
- External crowd platform – Exporting collected data and sending it to an external crowd-sourcing platform to process and label. This can improve scalability, but visibility of how crowd members are handling the data may be limited, and expertise in setting up questions for the crowd may be required.
- Integrated approach – Data collection and opinion mining happen seamlessly in the same platform. Crowd-sourcing process is managed and therefore requires less setup to initiate a project. Results may be accessible faster and can be analysed alongside the original data.
(Human) mind over matter
In addition to the humans’ cultural and social edge over machines, people are also better able to understand words and sentences with possible double meanings, and identify unclear objects of reference.
We’re are also better able to extract meaning in the face of unintentional ambiguity – misspellings, punctuation issues etc. – and may also be superior at gauging strength of sentiment.
Steeped in sarcasm – To a machine, the words proud and congrats indicate positive sentiment. To a human reader, however, it’s clear that the author’s true feelings are anything but positive. Examples such as this highlight the value of using a human integrated approach.
Drilling down to the drivers
Given that the most crucial work – isolating the specific drivers of sentiment, with fine discernment – is also the trickiest, human integration can be a valuable approach and provide the missing link when it comes to generating accurate opinion data.
Consider: 'The new phone is awful – hate the buttons!'
Here, the opinion expressed is multi-layered:
- Phone brand – negative
- Driver of sentiment – new products
- Specifics – keypad
Humans get humans and are able to provide context and understanding to complex conversations that pure AI struggles with currently. This approach can be applied to diverse topics including brand perceptions, market research and political issues.
Opinion mining at work
Understanding the drivers at work behind complex political outcomes has always been challenging.
For example, traditional polling techniques were unable to fully capture the dissatisfaction with the perceived establishment in the run-ups to both the 2016 EU referendum and US Presidential Election – and as a result failed to correctly predict the outcome of either. By simply analysing public conversations online, however, human-integrated opinion mining techniques were able to correctly anticipate the surprising outcomes of both. Why? The key factors driving public opinion – and underlying voter intent – were correctly identified. Many AI-pure approaches failed in the same respect.
As the world becomes more connected, differences between decision makers and their stakeholders are becoming more visible and volatile than ever. Traditional methods of understanding a broad group of people are breaking down because they can neither measure the intensity nor the commitment behind the emotions. Sophisticated analysis of online content in the form of opinion mining, however, offers a more reliable understanding of what is really happening in today’s world.
In the same way, opinion data can be used to power corporate decision-making whenever developing an accurate understanding of the drivers behind public or consumer behaviour is mission critical.
Human or crowd-integrated approaches remain necessary for drilling down into drivers behind opinion – and, hence, action. These approaches can be utilised not only when forming reactive strategies, but to anticipate future behaviour.
Using data responsibly: What opinion mining is and is not
Online privacy is a growing concern for many. So reading that online conversations are ‘mined’ might understandably cause some discomfort. Critically, opinion mining is not surveillance – nor is it profiling. Rather, it is a process of data aggregation that allows for better strategic decision making and experience improvement, based on insight into what groups of people feel and the factors driving their opinions. Responsible opinion mining keeps personal privacy paramount.
The future of opinion mining
Multinationals, media corporations, policy makers and research firms stand to benefit significantly from opinion mining. This is true for whoever seeks to develop a detailed, accurate understanding of public opinion – and, crucially, what is driving it. By tapping into the universe of unstructured opinion data, data that was previously unusable, these organisations are enabled to make better policy- and business-critical decisions.