
The intricacies of big data's Oscar predictions
Big data, big confusion. Any time data is at play, there’s a chance for confusion and misinterpretation, no matter how solid your algorithm. ‘Big data’ analytics companies - Cape Town-based Principa and New York-based Exponential - have both been using big data for years to predict the Best Picture winner at the annual Oscars. Unfortunately both predictions were incorrect this year – but that doesn’t mean we can’t learn from them.
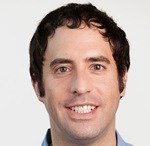
Bryan Melmed, vice president of insights at Exponential, says their system was designed to identify and understand consumers, but as the people who vote in the Academy Awards are so easily described, the system can be used to make a Best Picture prediction.
For example, Melmed shares that in 2012, the Los Angeles Times revealed that the Oscar voters were overwhelmingly Caucasian, overwhelmingly male, and with a median age of 62. Exponential then adds a job in the entertainment industry to these qualifiers, and the matching user profiles describe the preferences and behaviours for a small and distinct audience. So they don’t need to actually identify Academy members – membership is a secret, anyway – because finding people who live in the same circumstances is more than enough.
The next step in their process of predicting the Oscars’ big wins is to compare this group of Oscar voter lookalikes to the fan base for each of the Best Picture nominees. Whatever film offers the best alignment between the two is then Exponential’s prediction for Best Picture winner. Melmed clarifies that this step is necessary because they can’t discern the film preference of the Oscar lookalikes directly as the audience is just too small.
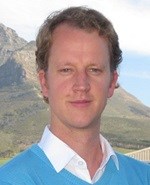
Thomas Maydon, head of data analytics at Principa, says they’ve been sourcing volumes of data from Oscar winners in the four major categories since 1935, which took 90% of their time. They then tested a few machine learning algorithms and chose a combination of algorithms that showed the greatest predictive power in sample and out of sample. This data was then added to their algorithms to identify patterns and trends and to determine what the best predictors and characteristics are of an Oscar winner. While they do look at diversity as a factor, Maydon says some of the best predictors identified have tended to be winning other awards, critics’ ratings and bookie odds, as well as genre and box office revenue before and after Oscar nominations.
Interestingly, Principa found that other awards ceremonies don’t always predict an Oscar win equally. On average, 62% of movies that won an Oscar for Best Picture also won the Golden Globe that year, whereas only 42% of movies won the Screen Actors Guild award. For the Best Actor category the numbers are 70% and 50% respectively, whilst for the Best Actress award the Golden Globe shoots ahead at 82% vs. 30%.
The Best Picture big surprise
That doesn’t mean it’s plainsailing though, as Melmed says it was really very difficult to pick between Spotlight and The Big Short. Fans of these films were very similar - their “audience DNA” matched 95% of the time. The main difference was that Spotlight was an older and more established audience, while The Big Short was more active in the film industry. In the digital marketing world, demographic information means very little but discounting it was Exponential’s downfall in choosing The Big Short.
Principa had similar issues. While the contest was very close, with The Revenant, Spotlight and The Big Short winning key awards in the lead-up to the Oscars as key predictors, Maydon says after crunching the numbers, their models indicated that Spotlight and The Revenant had an almost equal probability of winning, with less than 1% difference, “a virtual coin-toss”. Often, that’s what it comes down to. At least it proved correct in the other three categories for Principa: Best Actor, Actress and Director.
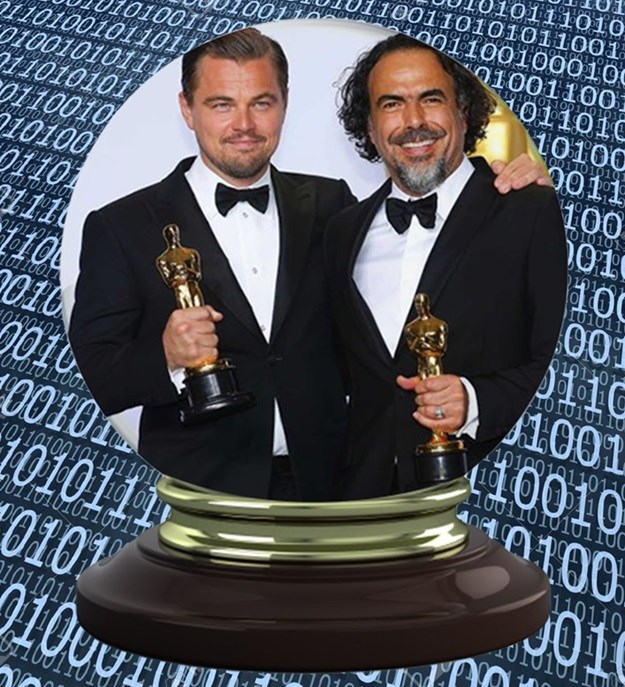
Melmed adds that if you’re an advertiser trying to identify your next customer, what people are reading about or thinking about is going to be far more predictive than what they look like. But many Academy voters have not worked in the entertainment industry for 10 or 20 years. If you try identify a group of people who did something 10 or 20 years ago, demographics will obviously be one of the few things that is still relevant, and actually far more important than Exponential realised – more important than living near Hollywood or even having an active interest in film.
Future predictions look cloudy
Melmed points out that as of next year, the Academy will have more restrictions on who is eligible to vote in an effort to shift the Academy to a younger, more diverse audience. That makes the outcome a lot less predictable, and their current data model obsolete.
While it’s been clear to Exponential for a while attribution models aren’t working, no one was sure if it was the models themselves or the data they rely on. Now that programmatic has exposed a lot of people to poor data quality, Melmed says the focus has shifted there, with people questioning if the data they are working with is unbiased and reliable – similar to what’s happening with Oscar voting.
Maydon agrees that there is plenty of information, thinking and great ideas out there about big data, innovative ways of using it, such as voice analytics in call centres, and new sources, such as wearable technology, to take advantage of and so on. More important though is getting the basics right and doing the necessary upfront thinking and planning and get the necessary buy-in from executives. “Ask yourself as a marketer first, ‘what answers do you need to make good decisions about marketing messages, targeting and determining ROI on marketing campaigns’,” recommends Maydon.
Melmed clarifies that this is nothing new, as: “every ‘human’ science, from medicine to sociology, has gone through a stage where a slew of old assumptions are re-evaluated and discarded. If marketing is going to be a science, we’re overdue to do this as well,” especially as he says big data marketing is sort of like the internet itself – “You can’t sit back and wait for something to come to you. It’s not television. But trust me, everything gets a lot more exciting once you start using it.”
Maydon adds that many companies already use customer data to predict future customer behaviour based on their profiles and past behaviour or on the past behaviour of individuals like them. If you understand customer behaviour on an individual profile level and are able to predict their actions with a reasonable level of accuracy using data, you can make decisions such as what product to offer them and even at what price to trigger an impulse buy, or whether to offer someone a loan, how much to offer and what interest rate to charge based on their profile and past behaviour.
It’s a truly fascinating field, but don’t just take Melmed and Maydon’s words for it: Click here for more on Exponential, and here for more on Principa.
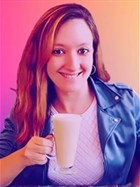
About Leigh Andrews
Leigh Andrews AKA the #MilkshakeQueen, is former Editor-in-Chief: Marketing & Media at Bizcommunity.com, with a passion for issues of diversity, inclusion and equality, and of course, gourmet food and drinks! She can be reached on Twitter at @Leigh_Andrews.