
Subscribe & Follow
Jobs
- Developer Graduate Remote
- Urban Futures Graduate Remote
- Data Science Graduate Remote
How AI and machine learning can help combat rhino poaching
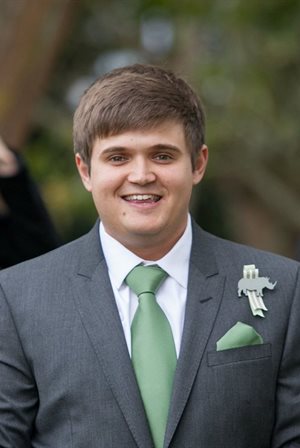
Le Roux completed the study under the supervision of Prof Thomas Niesler and Dr Riaan Wolhuter from SU’s Department of Electrical and Electronic Engineering. He conducted his research in the Digital Signal Processing Lab in the same department, where the project is still ongoing.
Passionate about nature conservation and the use of technology and artificial intelligence to solve some of our major challenges, Le Roux says he felt that there was need for technology that could assist nature conservationists in the fight against rhino poaching. He adds that since no single anti-poaching strategy can be used to fight this crime, various techniques should be combined to gain a strategic advantage.
Animal-borne automatic behaviour classification system
To make a contribution in this regard, Le Roux developed an animal-borne automatic behaviour classification system that can provide information about rhino behaviour in real time and assist with anti-poaching activities. Le Roux says conventional techniques for the classification of animal behaviour are performed offline as a post-processing step and does not provide real-time data analysis.
“To enable real-time analysis, data needs to be transmitted wirelessly to a receiver station and such transmissions are energy demanding. As a result of the resource limitations of current biotelemetry devices, behavioural studies are typically short-lived and range from days to weeks.”
In contrast, the system that Le Roux developed is a real-time machine learning model embedded in the microprocessor of a biotelemetry tag attached to an animal. He used these tags to collect data from rhinos at a private sanctuary.
“The tag monitors the output of a three-dimensional accelerometer (an instrument that measures the acceleration of a moving or vibrating body), after which it performs data pre-processing, feature extraction and subsequently classifies the sensor data into various behavioural classes, for example, standing, walking, running, grazing or lying down, before transmitting the result to a receiver station.
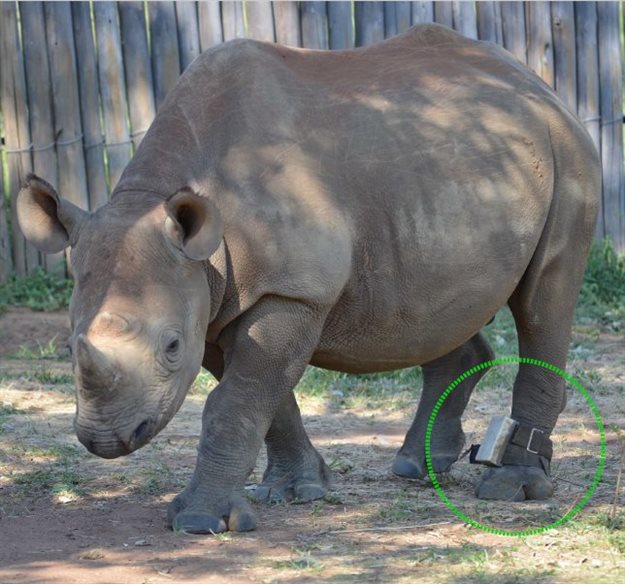
Abnormal behaviour detection
“The system also determines the animal’s location via a global positioning system (GPS) and, when combined with the behavioural information, provides valuable insights on what the animal is doing and where the animal displays specific behaviours.
“When attached to a rhino, the system provides updates every 6.5 seconds on where the rhino is and whether it is standing, walking or lying down.”
Le Roux adds that collecting such information over extended periods provides the opportunity to draw time-dependant heat-maps of animal behaviour, for example, where they tend to sleep, stand and walk.
“This would allow abnormal behaviour detection through questions such as ‘why is the rhinoceros lying longer than normal – is it injured, why is it suddenly walking in an unusual direction or why it is running?’
“A rhino’s behaviour is a key indicator of its stress levels and real-time updates can serve as an early warning of poaching.”
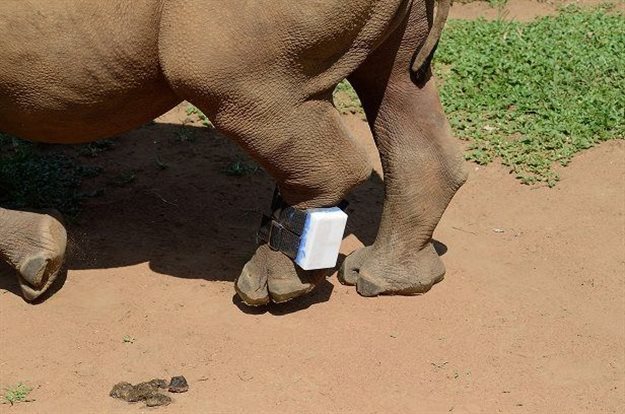
Improved biotelemetry tags
Le Roux says the biotelemetry tags used in his study improve on previous tags by utilising machine learning to process and subsequently discard the large volumes of raw sensor data and only transmitting the behavioural state and GPS location of the animal.
“The tags have an increased battery life and also consume 14 times less energy than conventional techniques, enabling long term deployment. This allows the probable real-time behaviour to be transmitted continuously, thereby providing an enhanced level of detail and immediacy.”
According to Le Roux, current biotelemetry tags are small and lightweight because they need to be attached to an animal of interest without harming the animal, while providing maximum comfort.
“As a consequence, the device must be powered by a small battery and necessitates the use of ultra-low-power microcontrollers that are limited in terms of available processing power and memory.” Le Roux adds that this can be problematic when performing studies on animal behaviour for extended periods.
He says his behaviour classification system can provide valuable information for nature conservationists, behavioural ecologists and farmers.
“The technique can also be applied to general embedded machine learning applications employed in smart phones, smart watches and sensors within the internet of things,” he adds.

