Take the SAM opportunity to optimise data management
As the Financial Services Board progresses through proposal stages with the forthcoming Solvency Assessment and Management (SAM) regulations, the opportunity is now ripe for insurance companies to design a pragmatic and sustainable data governance approach.
The goal of the SAM regime is to ensure that insurance firms carry adequate capital reserves to cover a crisis - such as a the relentless hailstorm that hit Joburg's East Rand last year, smashing the windows of thousands of houses and cars, and causing untold other damage. Like the Basel II legislation for banking, SAM requires that insurers prove that all risk calculations are based on quality data.
Looking more deeply into the subject shows clearly that the right approach can deliver benefits that extend well beyond the SAM regime's goal of minimising the requirement to hold excessive capital.
Typically, we see organisations veering too far towards one end of the spectrum - with some ensuring only the bare minimum of compliance and others taking an overly-rigorous approach that tries to govern too many elements and, therefore, fails to deliver business advantage.
The sweet spot
Companies such as the UK's Aspen Insurance have found that the sweet spot comes from implementing appropriate controls to identify and prioritise data issues that are exposing the business to risk (or have any other negative impact). Added to this, the controls serve to "future proof" the organisation so that as data governance regulations harden in the future, their processes easily evolve and keep pace with requirements.
The foundations of data governance start with a comprehensive analysis - asking questions like: What data are you using within the organisation? Where does the data come from? Which of the data is of the most critical importance? It is essential that across the organisation there is standardisation and that everyone is measuring data quality and importance on the same scales.
To arrive at this point, data stewards must identify and document business and data definitions, policies and rules. This can be a massive task, as the data stewards often battle with a variety of different data sources and analysis tools, as well as poorly understood processes, and organisational and people changes.
Relevant stakeholders from within the business must engage with data stewards to agree on new data elements, policies and business rules that are applicable to the entire organisation. The governance workflows emanating from this need to be established formally, with the buy-in from executives universally applied and aligned with business stakeholders.
Data dictionaries are documented - articulating the "consumption" and "production" of data, its materiality (how important to a given calculation), and the data quality rules and controls.
In Aspen Insurance's case, the Data Stewardship Platform helped reduce the workload on stewards, improved collaboration between stakeholders and reduced the maintenance burden by ensuring that critical documentation and artefacts are shared and kept current. The data governance organisation focusses data quality efforts to ensure maximum benefits.
A set of transparent processes
Ultimately, organisations will arrive at a set of transparent processes that monitor and measure the ever-changing risks to which they are exposed. For example, automated alerts for data quality issues are indexed to business priority or impact and actions are mapped in the governance policies that have been created.
All of this is what should happen in a perfect world - using best-of-class data quality technology to make the most of the process and ensure that the outcomes are sustainable. The unfortunate reality is that, too often, companies fail to address their data quality issues.
The consequences of a poorly conceived data quality strategy are manifold. On one hand, there is the probability that an insurer will have to maintain unnecessary liquidity - resulting in an opportunity loss as those funds remain unproductive. Having incorrect capital adequacy also often incurs regulatory penalties and diminished shareholder confidence.
Poor data quality, born out of systems errors, often causes a fundamental misunderstanding of an insurer's risk position. In one case, for example, a poorly documented change to how a system handed credit swap positions led to a downstream system interpreting all short positions as long positions. This led to a material mis-estimation of the firm's exposure.
The trend towards regulating and legislating data management requirements shows no sign of abating. The New Companies Act, the Consumer Protection Act (CPA) and the Protection of Personal Information (PoPI) Bill and the Foreign Account Tax Compliance Act (FATCA) are all examples of legislation that, like SAM, require more rigorous data governance and data quality.
Insurers that embrace data governance in a sensible way will gain the competitive advantage of reuse, flexibility and time to value over those that simply play lip service and have to start from the beginning for each new regulation.
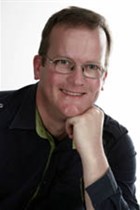