Five Big Data myths busted
This article debunks five common misconceptions that are creating confusion, and is supported by a comprehensive Big Data Analytics eBook that will give you an introductory approach to adopting big data analytics - what is the business case, what should you be thinking about, how should you approach the problem.
- Big data must be big
Every second big data presentation I see sprouts incomprehensible numbers at me. Yes, Hadoop can store data at a fraction of the cost of traditional Enterprise Data Warehouses (EDW). Most EDWs only store the data necessary to answer specific questions.
For example, if I want reports on the profitability of my online versus my 'bricks and mortar' stores, I may store data related to the answering of this question. If, a couple of years later, I am asked how many clients visited my web store and didn't buy anything, or bought from the traditional store later, I may not have stored the data necessary to answer this question. Hadoop allows us to store more data - data that we may need some day but don't necessarily know that we need now.
However, the real value of big data is the ability to bring together structured and unstructured data and analyse this very quickly. For example, I may want to bring together data from my Google Ads, my web logs, my online store system and my EDW in order to answer the "client visited but did not buy" question. I need this information quickly so that I can make the browsing client, who I expect to lose, a special offer while they are still online. This cannot be easily achieved with the EDW but is relatively simple to do using big data.
Use cases such as these may not require vast amounts of data. Rather they require the ability to bring together both structured and unstructured data to answer the question.
- Big Data is about social media
Social media and big data have been sharing a lot of press, leading many people to believe that big data is all about social analytics. While social media sources, such as Twitter and Facebook, can be used for big data analytics, very few existing adopters are focussing here.
Rather, most use cases focus on using existing data sources more effectively. Traditional EDW approaches rely on highly structured schemas (database designs) and complex Extract, Transform, Load (ETL) processes that are time consuming and expensive to adapt. By comparison, big data approaches are quick and cheap. Big data storage is also much cheaper than the EDW because solutions such as Hadoop leverage cheap, commodity hardware.
Big data can be used to optimise the existing data warehouse, or act as a 'sand box' environment to allow business users to "test a theory" before asking the data warehouse team to develop it formally.
- Big data will replace the existing EDW
The enterprise data warehouse plays an important role in supporting enterprise reporting and "slice and dice" Business Intelligence (BI) that will not be replaced by a big data solution. These BI solutions use structured data and lead to reports that aggregate or summarise that data. The EDW provides data models that allow a variety of known questions to be asked of the data.
On the other hand, big data uses cases work with data that is of high complexity - where both the type and volumes of data may be changing frequently. In most cases, they allow business to ask questions that they may not have previously been able to ask - with the goal of creating actionable insight.
In most uses cases, for example, customer segmentation or value mapping, the EDW becomes a source to the big data analytics engine, where it is combined with additional sources. The big data platform performs advanced analytics and the results may be transferred back to the EDW to become a source for standard BI reports.
Big data is a complementary solution to most existing BI solutions.
- The biggest challenge for big data is handling volume
Big data implies large volumes, and, depending on the use case, may well require large volumes. Yet, large EDW solutions handle large volumes reasonably successfully, as long as the data sources are structured and fit into existing schemas.
Data integration is a far bigger challenge than volume. With thousands of data sources, ranging from web and system logs, to social media feeds, to existing CRM and EDW applications, or even machine data feeds, big data integration is complex. Traditional ETL tools and Structured Query Language (SQL) based data bases simply cannot cope. The technical staff that rely on these existing skills cannot necessarily cope either.
In fact, the biggest challenge for big data is a lack of skills and time. Most organisations have an existing pool of skilled EDW developers, SQL programmers and the like.
The challenges of integrating disparate big data sources and performing relevant predictive analytics on them are new to most companies. Training existing staff in predictive analytics and similar skills is clearly an option.
But traditional build approaches to big data analytics still take a long time and depend on expensive technical resources, maybe even external consultants. Business cannot afford to wait years when competitors are acting on improved insights now.
Self-service big data platforms, such as Datameer, give business analysts and management the ability to integrate and analyse complex data sets within weeks or months, without a dependency on expensive and scarce technical resources. Datameer allows you to focus on the questions you need answered to run your business, rather than on the technology needed to answer the questions.
- Big data is just hype - there are no practical applications
Big data is not just another BI application. In fact, most successful use cases for big data complement existing BI solutions. However, big data is not required in all cases, and, should not be seriously considered without a decent use case.
So where are early adopters getting their successes?
There are clear returns for organisations looking to optimise their existing data warehouse. Here the business case is driven by the ability to store more data, to integrate disparate data sources quickly, and to develop this more quickly than traditional, rigorous EDW approaches. Another common IT use case is to identify network failures and other issues before they become serious - improving operational efficiency by reducing downtime on critical systems.
Other big data use cases tend to favour particular industries. Retailers and financial services companies are offering an improved customer experience and maximising profits by using big data analytics to improve customer segmentation, optimise prices or reduce fraud. Telecommunications companies are able to better predict network capacity, saving hundreds of millions in infrastructure costs. In government, big data analytics helps to increase revenue collection and identify security threats.
If you are unable to meet your existing analytics needs quickly enough, or at all, with your existing BI solution then a big data analytics platform may be what you need.
Download the big data analytics ebook to find out more about big data.
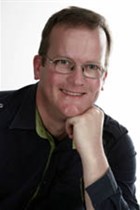