Four steps to data transformation

Data transformation is a relatively mundane yet fundamental data management capability – particularly when dealing with similar data from multiple sources. Here are three simple examples:
- System A represents Male and Female as 0 and 1, while System B represents Male and Female as M and F
- System A stores dates in the US format (MM/DD/YYYY) whilst System B uses the South African format (DD/MM/YYYY)
- System A uses a comma as a decimal point (123,33) while System B uses a point (123.33)
In each case, we need to transform the data into a common format for aggregating, matching and reporting. There are four crucial steps that can significantly assist with any data transformation process. Syncsort’s ETL (Extract, Transform and Load) and CDC (Change Data Capture) capabilities are particularly highly rated by analysts based on their ability to transform data from diverse formats e.g. when moving data from the mainframe to relational databases and Hadoop. Adding Syncsort Trillium Software to the mix ensures award-winning data profiling, cleansing and matching across these platforms.
The four steps to data transformation
Step 1: Data interpretationBefore we can begin any transformation process, we need to understand what our data looks like. This is most efficiently done using data profiling tools.
Step 2: Pre-translation quality checkAt this step, we validate data against known requirements and standards to identify any errors or issues that we may not expect and must address.
Step 3: Data translationThis is the physical process of changing data into the required format. Interestingly, we may not only change the content of a data set, but also the format such as converting a CSV file to an XML format.
Step 4: Post translation quality checkWe repeat step two at after running our translation to measure the improvement and test the outcome. By planning for and following these four steps you should quickly begin to improve the usefulness of your aggregated data.
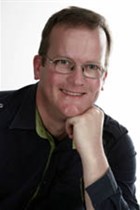
About Gary Allemann
MD of Master Data Management He is passionate about Information Communication Technology (ICT) and more specifically data quality, data management and data governance.